What is Generative AI? Unveiling the Future of Creative Technology
Published Monday, February 26, 2024 By TechRant Staff
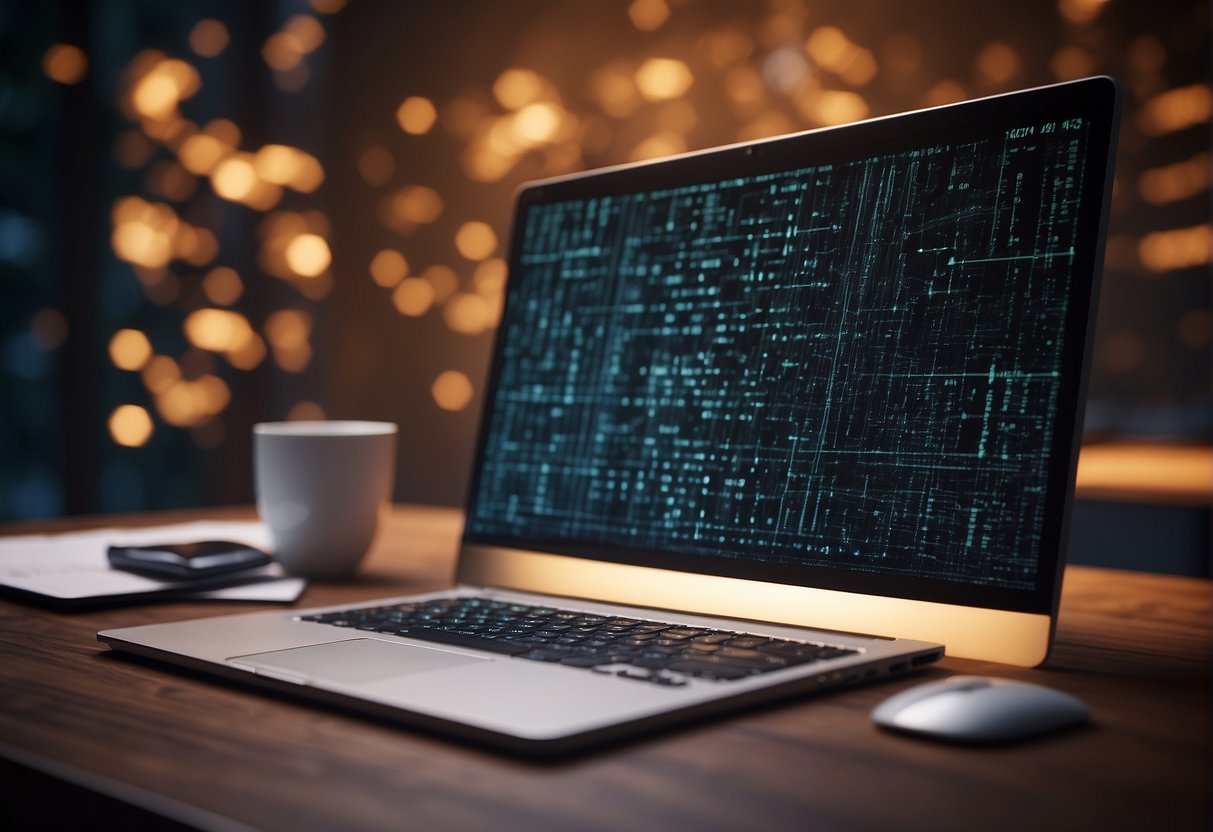
Generative AI stands at the forefront of technological progress in the field of artificial intelligence, representing a paradigm shift in the way machines understand and create content. This technology relies on sophisticated algorithms capable of producing new, original materials such as text, images, audio, and code. The core principle enabling this functionality is the use of generative models which, when fed a prompt or data input, can generate outputs that mimic human-like creativity.
As a derivative of artificial intelligence, generative AI has the potential to transform a multitude of industries through its capability to automate creative tasks. Extensive research has propelled this technology into various applications, ranging from the generation of realistic images to the creation of music and textual content. It serves not only as a tool for enhancing productivity but also as a means of pushing the boundaries of AI capabilities by teaching machines to interpret context and generate relevant, sophisticated responses.
The development of generative AI involves rigorous cycles of training and learning, wherein models are exposed to large amounts of data, enabling them to learn patterns, styles, and structures. This allows the generative AI to produce outputs that are not just random, but purposeful and context-aware. As this technology evolves, it would continually reshape the landscape of what is achievable with artificial intelligence, bridging the gap between human and machine creativity.
Core Concepts of Generative AI
Generative AI revolves around the premise of creating new, original data resembling the training inputs. Its advanced models interpret and learn from data to generate similar patterns.
Understanding Generative Models
Generative models are designed to understand and replicate the distribution of data they learn from. These models capture the intricacies of data in such a way that they can generate new instances that could be mistaken for real data. Key components include:
- Neural Networks: They are the backbone of generative AI, providing the architecture to learn from complex datasets.
- Variational Autoencoders (VAEs) and Generative Adversarial Networks (GANs): VAEs are known for their proficiency in producing high-quality results by encoding inputs into a latent space, whereas GANs involve a duo of networks contesting with each other to generate new data points.
Popular Generative AI Technologies
Several generative AI technologies have emerged as leaders in the field:
- GPT-3 and ChatGPT: These are based on the transformer architecture and are designed to understand and generate human-like text.
- DALL-E: Excels in creating images from textual descriptions, showcasing the model’s ability to cross the boundary between text and visual content.
- BERT: It enhances language understanding and helps in generating text that can be contextually relevant in smaller fragments.
Generative AI in Machine Learning and Deep Learning
In the realms of machine learning and deep learning, generative AI has become a cornerstone:
- Foundation Models: These large-scale models, such as GPT-3, serve multiple purposes and applications, stemming from their extensive training over diverse data sets.
- LLMs (Large Language Models): They have significantly contributed to advancements in various language-related tasks and continue to evolve, pushing the boundaries of what AI can achieve in terms of human-like text generation.